Identification of Plant Leaf Disease Using a Novel Convolutional Neural Network
DOI:
https://doi.org/10.48001/jofsn.2023.1111-15Keywords:
Convolutional Neural Network (CNN), Dataset, Image processing, Plant leaf, Signal processingAbstract
A critical element in preventing a major outbreak is the detection of plant leaves. An important research issue is the automatic detection of plant diseases. For both human life and condition, a plant's dedication is essential. Like humans and other animals, plants do suffer the negative impacts of illnesses. A plant's normal development is influenced by the frequency of plant diseases that occur. The entire plant, including the leaf, stem, organic material, root, and flower, is affected by these diseases. Most of the time, if a plant's ailment is not treated, it dies or may cause leaves, blooms, organic products, and so forth to fall off. For accurate identification and treatment of plant diseases, appropriate determination of these disorders is necessary. Plant pathology is the study of plant infections, their causes, and methods for preventing, managing, and eradicating them. However, the current approach includes human inclusion for structure and identifying disease proof. This tactic is time-consuming and expensive. Instead of using the current method, a programmed division of diseases from plant leaf images utilising a delicate registration methodology may be more beneficial. In this study, we describe a method for identifying and characterising plant leaf diseases naturally called Bacterial Searching Improvement Based Radial Basis Function Neural Network (BRBFNN). We use bacterial search streamlining (BFO), which increases the speed and accuracy of the system to recognise and organise the regions contaminated by diverse illnesses on the plant leaves, to assign Radial Basis Function Neural Network (RBFNN) the proper weight. The location development calculation increases the system's efficiency by searching for and gathering seed focuses on typical traits for the highlighted extraction operation. To make progress against parasite diseases including early curse, leaf twist, leaf spot, late scourge, and basic, cedar apple, and leaf rust. The suggested approach achieves more accuracy in identifying evidence and characterizing infections.
Downloads
References
Agarwal, G., Belhumeur, P., Feiner, S., Jacobs, D., Kress, W. J., Ramamoorthi, R., ... & White, S. (2006). First steps toward an electronic field guide for plants. Taxon, 55(3), 597-610. https://doi.org/10.2307/25065637.
Du, J. X., Wang, X. F., & Zhang, G. J. (2007). Leaf shape-based plant species recognition. Applied Mathematics and Computation, 185(2), 883-893. https://doi.org/10.1016/j.amc.2006.07.072.
Jora, R. B., Sodhi, K. K., Mittal, P., & Saxena, P. (2022, March). Role of artificial intelligence (AI) in meeting diversity, equality, and inclusion (DEI) goals. In 2022 8th International Conference on Advanced Computing and Communication Systems (ICACCS) (Vol. 1, pp. 1687-1690). IEEE. https://doi.org/10.1109/ICACCS54159.2022.9785266.
Mittal, P., Jora, R. B., Sodhi, K. K., & Saxena, P. (2023, March). A review of the role of artificial intelligence in employee engagement. In 2023 9th International Conference on Advanced Computing and Communication Systems (ICACCS) (Vol. 1, pp. 2502-2506). IEEE. https://doi.org/10.1109/ICACCS57279.2023.10112957.
Neto, J. C., Meyer, G. E., Jones, D. D., & Samal, A. K. (2006). Plant species identification using Elliptic Fourier leaf shape analysis. Computers and Electronics in Agriculture, 50(2), 121-134. https://doi.org/10.1016/j.compag.2005.09.004. Park, J., Hwang, E., & Nam, Y. (2008). Utilizing venation features for efficient leaf image retrieval. Journal of Systems and Software, 81(1), 71-82.
https://doi.org/10.1016/j.jss.2007.05.001.
Wang, X. F., Huang, D. S., Du, J. X., Xu, H., & Heutte, L. (2008). Classification of plant leaf images with complicated background. Applied Mathematics and Computation, 205(2), 916-926. https://doi.org/10.1016/j.amc.2008.05.108.
White, S. M., Marino, D., & Feiner, S. (2007, April). Designing a mobile user interface for automated species identification. In Proceedings of the SIGCHI Conference on Human Factors in Computing Systems (pp. 291-294). https://doi.org/10.1145/1240624.1240672.
Downloads
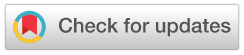